Data is the business asset for every organisation which is audited and protected. Data can be any form such as structured, semi-structured and unstructured. To handle any kind of the data, Data Lake comes in the picture as a centralized repository to store the data as-is (relational data from line of business applications, and non-relational data from mobile apps, IoT devices, and social media). The types of raw data that are stored in a data lake can include:
- Audio, images and video
- Communications (blogs, emails, social media, click-streams)
- Operational data (inventory, sales, tickets, tourism)
- Machine-generated data (log files, IoT sensor readings)
The most importantly, data lakes are specifically designed to run large scale analytics workloads in a cost-effective way. Within Data Lake, the necessary data is made available to all levels of employees, irrespective of their level or the designation.
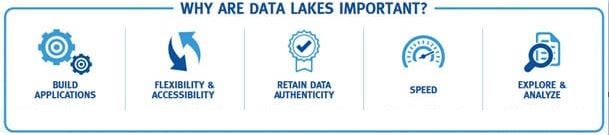
Essential Elements of a Data Lake are:
Data Lake Analytics allow various roles in your organization like data scientists, data developers, and business analysts to access data with their choice of analytic tools and frameworks.
Data movement & Governance such as moving data analytics to the source , the data lake and the edge. An interesting development in this sense is that you see the applications (or big data analytics) moving to the edge rather than to a storage repository to move even faster and take away the burden from networks, among others.
Security, Data Quality and Storage in a data lake allows to store relational data like operational databases and data from line of business applications, and non-relational data like mobile apps, IoT devices, and social media. Stored data doesn’t need to be moved or transformed before you perform data analysis, and the total cost of ownership is further lowered because of the hierarchical namespace of stored data.
Data lakes are highly scalable and flexible. That doesn’t need too much elaboration. The system and processes can easily be scaled to deal with ever more data. Data quality is a necessary condition for consumers to get business value out of the lake.
Machine Learning run real-time analytics and machine learning to your data to produce better, actionable insights including reporting on historical data, and doing machine learning where models are built to forecast likely outcomes, and suggest a range of prescribed actions to achieve the optimal result.
No comments:
Post a Comment